Datalearning 2023: CyclingOracle AI-model
Thursday 14 December 2023 • Stats
CyclingOracle's AI-model predicts the winner of all cycling races for men and women. We are constantly trying to improve model. In this blog you will find some background to the model, an explanation of the predictions and the idea behind a number of improvements.
As cycling enthusiasts we are looking forward to the upcoming races. CyclingOracle is there for the cycling fan who, just like us, speculates with his friends about the course of the next race and discusses the biggest contenders. With the rider-cards and predictions of the AI model, we give you all the ingredients for a good chat, a sharp discussion and great joy.
CyclingOracle's AI model predicts the winners of races based on the qualities of the riders. The model is based on the results of riders over the past 3 years, with recent results given more weight. These results are put into the model for all riders and for all UCI races: this produces an immense amount of data. The result of a race, in combination with the strength of the field of participants, the UCI classification and the altitude profile, together ensure that a rider scores points. A rider can score points from 20 to 99 on no fewer than 13 different skills, namely sprint, flat, mountain, hill, time trial, long time trial, short time trial, prologue, cobblestones, lead-out, general classification, one-day race and stage in a stage-race. In addition, a rider receives form-points for a recent good result.
How does the AI model work?
Each race awards points for a number of these 13 indicators, depending on the type of competition, altitude profile and the result. A bunchsprint awards points to riders in a different way than a time trial or a race that is won solo. A victory in a monument is assessed differently than a stagewin in a 2.1 race. In this way, the AI-model weighs the severity and importance of the race and therewith the performance of the rider.
We won't bore you with formulas, but here is an example: If a rider achieves a high ranking in a race with an uphill finish, he will receive points for 'Mountain'. If he or she beats riders with many good results in mountain races, he or she will receive a bonus, depending on the number strong climbers at the start - and in the top-10 of the race. The stronger the climbers' starting field and the stronger the climbers in the top 10, the more bonus points a rider can score. The amount of those bonus points depends on the rider's score on the relevant indicator. If a rider wins who has not often shown to be a great climber, she or he will receive a larger bonus than the already best climbers in the peloton would receive.
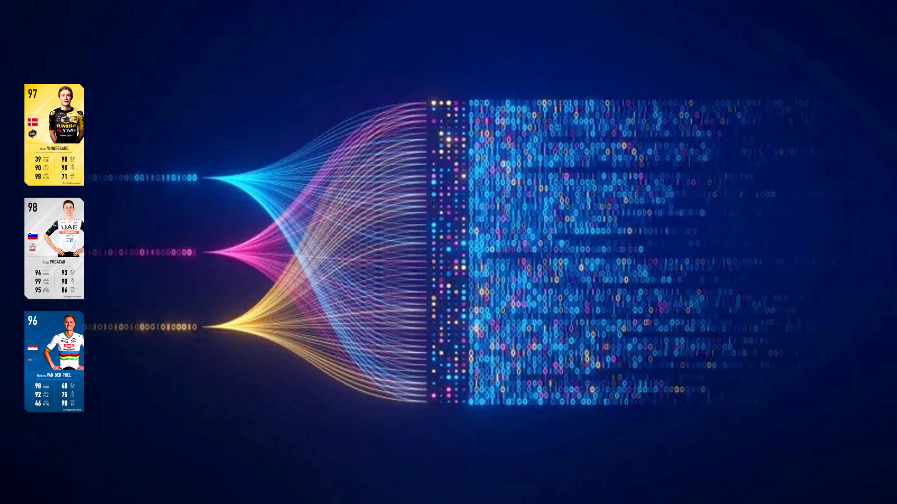
From rider qualities to prediction
We are not yet done after classifying the qualities of the riders. These indicators allow the AI model to apply them to races and predict them. All 13 qualities of the rider are used to determine how likely he or she is to win a race. Based on the field of participants, the type of race and the altitude profile of the next race, the AI model calculates an 'Expected Win (xW)' for each rider. In addition to the scores on the 13 specialties, the model also takes into account the rider's form, which is particularly important in one-week stage races and Grand Tours.
An important note about the predictions of the AI model is that they are limited to publicly available data that can be objectively attributed to races and riders. Information about injuries, attitude of riders (domestiques for a teammate or riding in preparation for later goals), weather and crashes is not of sufficient quality and can therefore not be usefully included in the model.
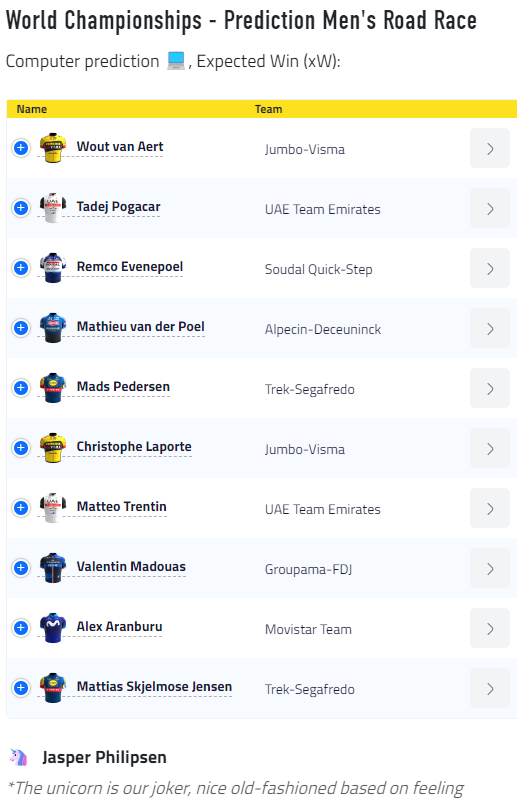
How did we improve the AI-model?
The AI model worked very well last season and regularly correctly predicted the winners of races. Yet as CyclingOracle we are always looking for improvements. And three have been found!
Sprint bonus! If a race doesn't include any significant hills, mountains or cobblestones, there's a good chance it will end in a sprint. However, not all flat races are decided in a bunchsprint. That is why the model also looks at time differences and the size of groups at the finish in this type of races. The bonus that riders receive for 'Sprint' now also depends on the course of the race and the result. Sprinters also receive more points if they perform well in bunch sprints in major competitions (e.g. Tour de France) and against the strongest competitors.
Starting field bonus. The way the AI model awards bonuses for competing against the best riders in the world has been improved and tailored to the strongest possible startlist. In addition, the personal bonuses are made dependent on the qualities of the riders themselves in the relevant specializations. If you beat riders who have been better in recent years, you score a higher bonus.
Point scale. Finally, in the predictions it became clear that smaller classics sometimes made a larger contribution to a rider's qualities than the most important races. The point scales of races have been optimized based on the importance of the race.
Questions or thoughts? Please!
Hopefully this helps to understand better how the rider-cards are created and the quality of riders is determined in order to subsequently predict the results of cycling races. As mentioned, we are constantly trying to improve the AI-model using new information, sources and insights. If you have any questions or suggestions, we are open to constructive ideas and contributions. You can email us at [email protected].